RadGS-Reg: Registering Spine CT with Biplanar X-rays via Joint 3D Radiative Gaussians Reconstruction and 3D/3D Registration
First Author*, Second Author*, Third Author
Institution Name Conferance name and year *Indicates Equal Contribution
Paper Supplementary Code arXiv
Abstract
Computed Tomography (CT)/X-ray registration in image-guided navigation remains challenging because of its stringent requirements for high accuracy and real-time performance. Traditional “render and compare” methods, relying on iterative projection and comparison, suffer from spatial information loss and domain gap. 3D reconstruction from biplanar X-rays supplements spatial and shape information for 2D/3D registration, but current methods are limited by dense-view requirements and struggles with noisy X-rays. To address these limitations, we introduce RadGS-Reg, a novel framework for vertebral-level CT/X-ray registration through joint 3D Radiative Gaussians (RadGS) reconstruction and 3D/3D registration. Specifically, our biplanar X-rays vertebral RadGS reconstruction module explores learning-based RadGS reconstruction method with a Counterfactual Attention Learning (CAL) mechanism, focusing on vertebral regions in noisy X-rays. Additionally, a patient-specific pre-training strategy progressively adapts the RadGS-Reg from simulated to real data while simultaneously learning vertebral shape prior knowledge. Experiments on in-house datasets demonstrate the state-of-the-art performance for both tasks, surpassing existing methods.
Pipeline
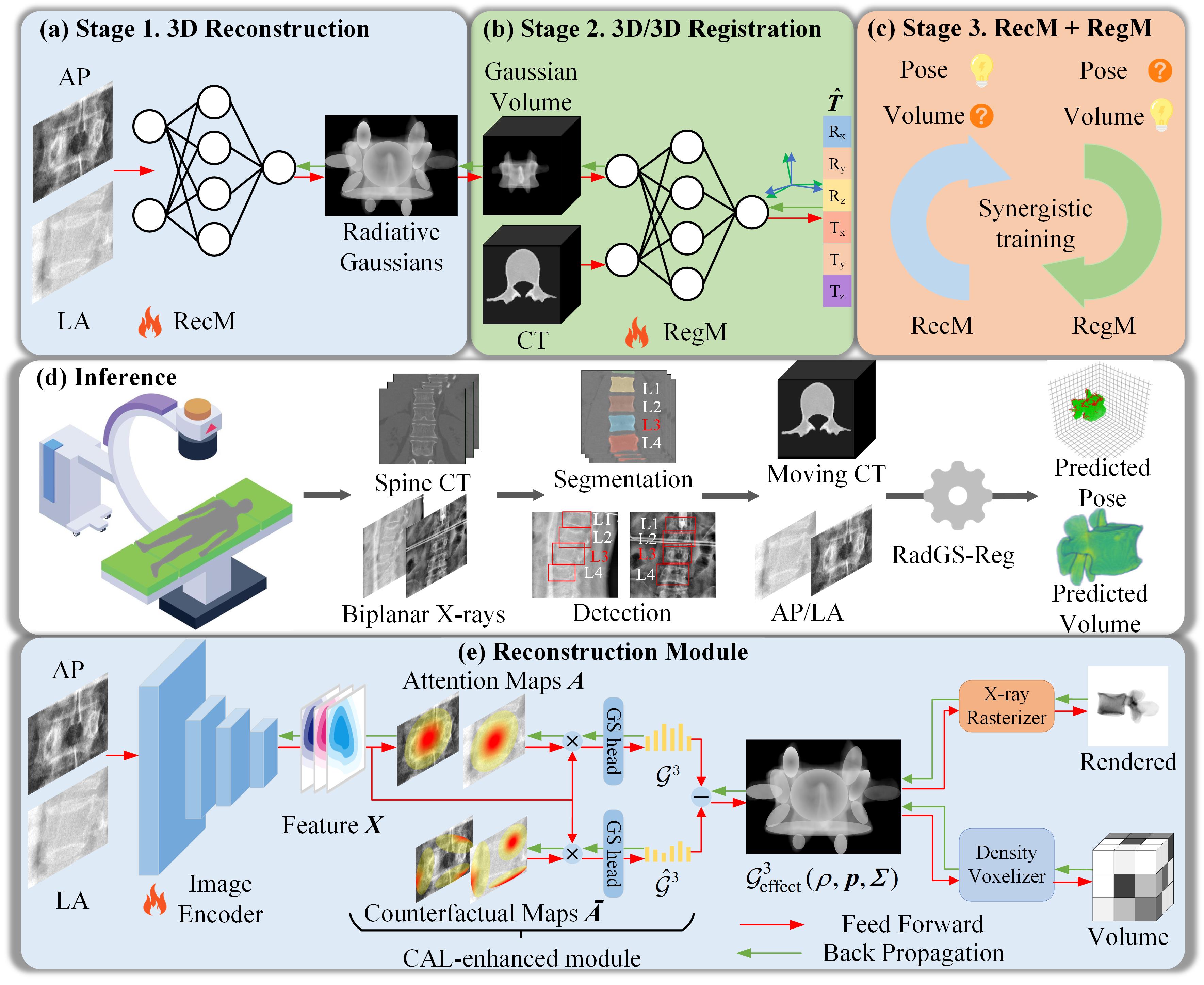
Qualitative Results
Reconstruction Results
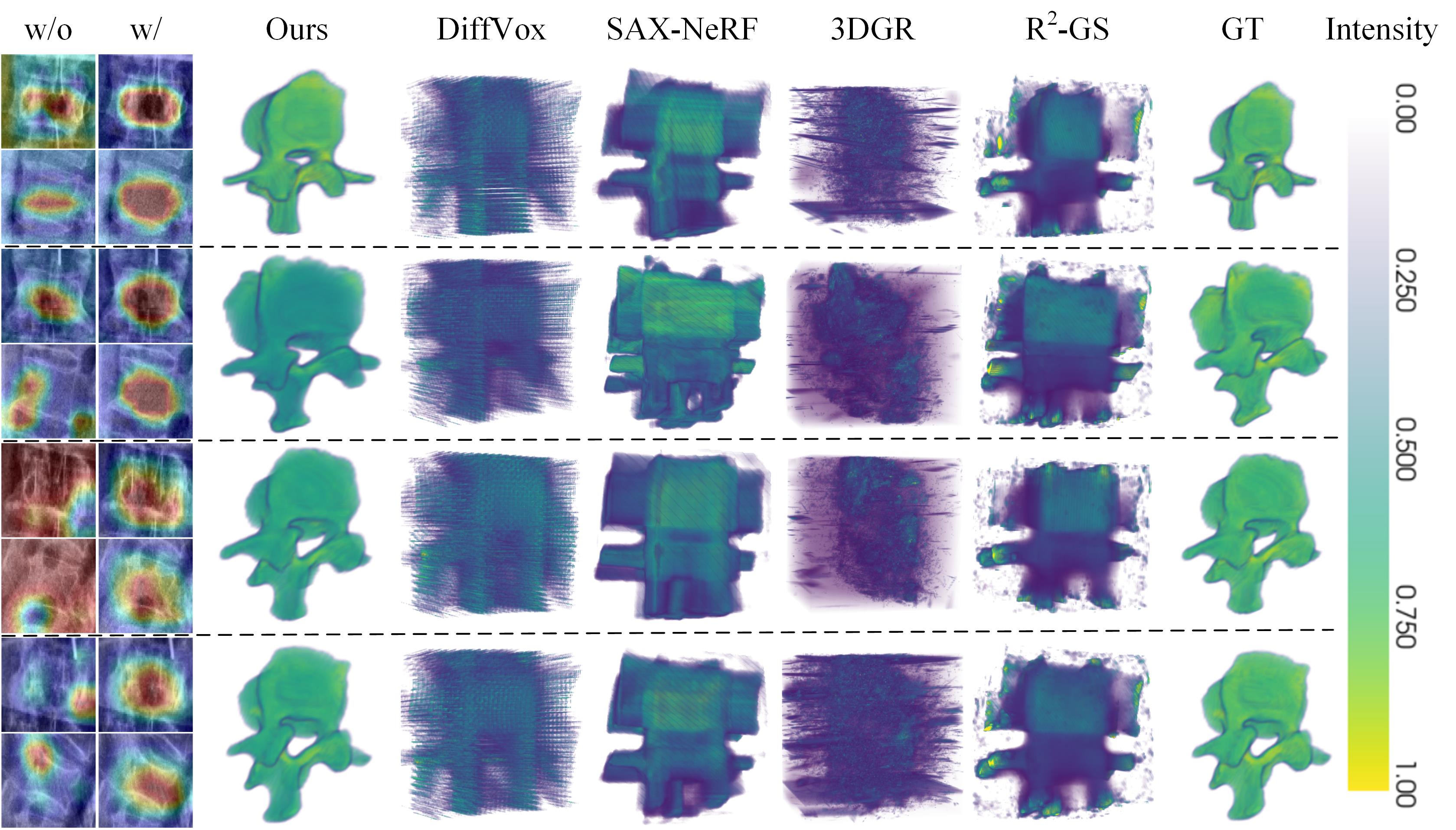
Registration Results
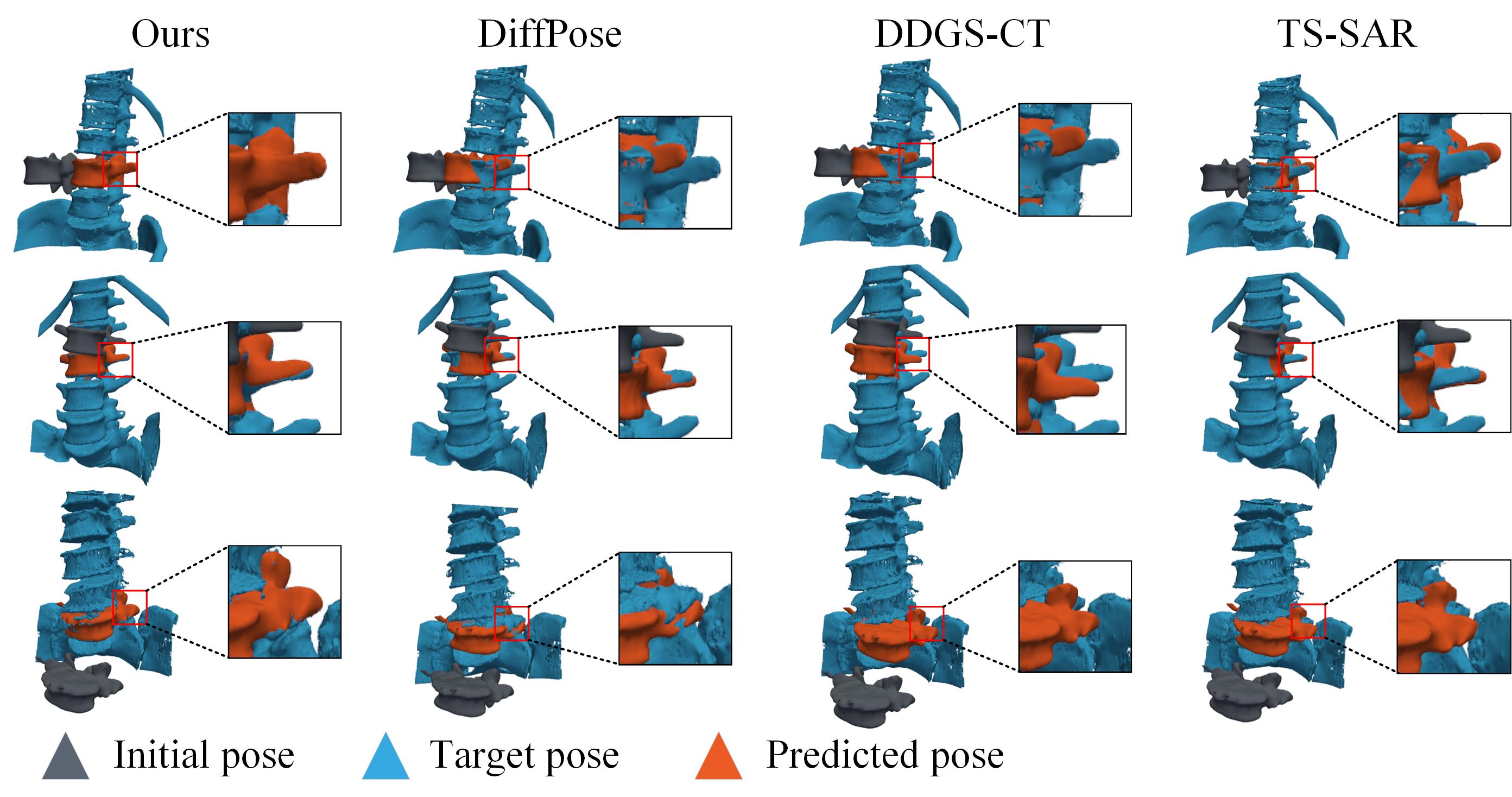